Efforts to predict natural disasters with precision have long been a pursuit of the scientific community, yet the elusive nature of these catastrophic events challenges even our most sophisticated technologies. While advancements in AI and data analysis have shown promise, the unpredictable complexities of natural systems still leave room for doubt. The question of whether we can truly forecast natural disasters accurately remains a contentious topic, with implications that extend far beyond our current understanding.
Key Takeaways
- AI enhances earthquake prediction accuracy through advanced seismic data analysis.
- Data accuracy challenges impact flood prediction reliability and effectiveness.
- AI technologies improve volcanic eruption forecasting precision and timeliness.
- Overcoming challenges in natural disaster prediction requires ongoing technological advancements.
Importance of Predicting Natural Disasters

The accurate prediction of natural disasters plays a crucial role in mitigating human casualties and minimizing property damage, making it a cornerstone of effective disaster management strategies. Accurate prediction allows for early warnings to be issued, enabling timely evacuation plans and preparedness measures to be put in place. Lives can be saved as people have more time to move to safety, and property damage can be reduced significantly. Advanced AI systems have revolutionized the field of disaster prediction, enhancing the precision and reliability of forecasts. By leveraging these technologies, authorities can allocate resources more efficiently for disaster response, ensuring that help reaches those in need promptly. Moreover, reliable predictions foster better communication and coordination among government agencies and communities, facilitating a more cohesive and organized approach to disaster management. In essence, the ability to predict natural disasters accurately is essential for safeguarding lives and minimizing the impact of such events on both people and property.
Advancements in Earthquake Prediction
Current advancements in earthquake prediction rely heavily on AI systems that analyze seismic data to forecast earthquake magnitude and patterns with increasing accuracy. Deep learning technologies have significantly enhanced the precision of earthquake prediction models, leading to more reliable forecasts. Initiatives by prominent institutions such as Google and Harvard are dedicated to further improving aftershock prediction precision, while countries like Japan utilize satellite monitoring to effectively predict earthquakes and tsunamis.
Seismic Activity Monitoring
Utilizing advanced AI systems and deep learning models, seismic activity monitoring has significantly enhanced the accuracy of earthquake prediction. By analyzing seismic data and identifying patterns through deep learning models, scientists can better predict earthquake occurrences. Satellite monitoring coupled with AI technology enables the detection of structural deformations, aiding in the mitigation of potential earthquake damage. Leading research institutions, such as Harvard, are exploring initiatives to improve aftershock prediction accuracy using AI-based approaches. Countries like Japan leverage technologies like satellites for earthquake and tsunami prediction by monitoring earth images. These advancements in seismic activity monitoring showcase the potential for more precise and timely earthquake predictions, contributing to better disaster preparedness and mitigation strategies.
Data Analysis Techniques
Advancements in earthquake prediction through data analysis techniques have revolutionized the field of seismic activity monitoring, paving the way for more accurate and timely forecasts of natural disasters. Deep learning systems analyze seismic data patterns to predict earthquakes accurately, while AI applications enhance earthquake prediction by studying magnitude variations. Additionally, the use of satellite imagery alongside AI models has contributed to improved earthquake aftershock forecasts. By leveraging AI to detect structural deformations and aid in developing effective mitigation strategies for earthquake risks, the scientific community is making significant strides in better understanding and preparing for seismic events.
Limitations in Earthquake Prediction

Predicting earthquakes accurately poses a formidable challenge due to the intricate nature of fault systems and the absence of definitive precursors. The limitations of current methods in pinpointing the precise timing and magnitude of seismic events underscore the complexities involved in earthquake forecasting. To enhance predictive capabilities, advancements in technology are crucial to overcome the hurdles posed by seismic activity patterns and data accuracy issues.
Seismic Activity Patterns
Amid the intricate web of seismic activity patterns lies a formidable challenge in accurately predicting earthquakes. AI models play a crucial role in analyzing seismic data to enhance earthquake prediction accuracy by identifying earthquake magnitude and patterns. However, the complexity of seismic activity, which includes various factors like fault lines, plate movements, and underground structures, poses significant hurdles in achieving precise forecasts. Understanding seismic activity patterns is vital not only for predicting earthquake occurrences but also for anticipating potential aftershocks. While AI advancements offer promise in improving earthquake forecasting, the limitations in prediction underscore the necessity for the continued development of sophisticated algorithms to decipher the complexities of seismic behavior.
Data Accuracy Challenges
Given the inherent complexities within seismic data interpretation and the challenges associated with sensor signals, the accuracy of earthquake predictions is often hindered by limitations in data accuracy. Interpreting data patterns and signals from sensors is crucial for understanding seismic activities, yet it poses significant challenges due to the dynamic nature of fault behavior and geological structures. Inaccuracies in data quality and gaps in monitoring networks further impede the precision of earthquake forecasts. Uncertainties in tectonic processes add another layer of complexity, making it challenging to develop reliable prediction models. Addressing these data accuracy challenges is essential for enhancing the effectiveness of earthquake predictions and improving preparedness for potential disasters.
Technology Advancements Needed
The challenges posed by the complexity and unpredictability of seismic events underscore the critical need for technological advancements in earthquake prediction.
- Improving seismic monitoring technologies for enhanced data collection.
- Advancing understanding of fault dynamics to predict earthquake behavior more accurately.
- Enhancing early warning systems and seismic networks for quicker and more reliable alerts.
Current technology struggles to accurately forecast the timing, magnitude, and location of earthquakes due to limitations in data accuracy and fault dynamics comprehension. Scientific advancements are crucial to refining seismic monitoring and modeling, which will ultimately lead to more precise earthquake predictions. By investing in technology enhancements and scientific progress, the accuracy of earthquake forecasting can be significantly improved.
Forecasting Flood Events

Utilizing advanced artificial intelligence technologies, the accurate forecasting of flood events has become increasingly achievable through the analysis of rainfall records and climate data. AI platforms, such as those being developed by Google for flood prediction in India, rely on flood simulations to enhance accuracy. Urban flood monitoring has benefited from a combination of AI technology and crowd-sourced data, enabling timely alerts to consumers through platforms like Google Maps. Deep learning applications within AI systems further improve flood prediction accuracy, aiding in effective disaster management strategies. By harnessing the power of AI to interpret vast amounts of data, including rainfall patterns and climatic conditions, the potential for more precise and timely flood predictions is steadily increasing. These advancements mark significant progress in mitigating the impact of floods on communities and enhancing overall resilience to natural disasters.
Challenges in Flood Prediction
The accuracy of flood prediction models is often compromised by challenges in data availability and the intricate nature of factors influencing flooding events. Model complexity issues further exacerbate the difficulty in accurately forecasting flood events, particularly in regions with limited data. Addressing these challenges is crucial for enhancing the effectiveness of flood prediction strategies and mitigating the impacts of unpredictable flooding events.
Data Accuracy Challenges
Limited data accuracy poses a significant challenge in effectively predicting floods, potentially compromising the reliability of AI models designed for this purpose. Inaccurate input data can lead to flawed flood predictions, impacting the performance of AI systems. Addressing data accuracy challenges is vital for improving the precision of flood predictions.
- Challenges in flood prediction include limited data accuracy in some regions, hindering the effectiveness of AI models.
- Inaccurate input data can lead to flawed flood predictions, impacting the reliability of AI systems.
- The quality of data collection plays a crucial role in addressing challenges in flood prediction accuracy.
Model Complexity Issues
Navigating the intricate landscape of flood prediction poses challenges particularly in managing the complexity of models essential for accurate forecasting. Complex models in flood prediction, incorporating artificial intelligence (AI) and machine learning techniques, demand extensive computational resources. However, balancing model complexity with real-time monitoring needs is critical to ensure timely warnings. The use of advanced algorithms helps optimize predictions under varying environmental conditions. High model complexity can lead to longer prediction times, impacting the effectiveness of early warning systems. To enhance accuracy, models must efficiently process seismic data and adapt to changing environmental factors. Addressing model complexity issues remains pivotal in improving the reliability of flood predictions and mitigating potential disasters.
Predicting Volcanic Eruptions

Utilizing advanced AI technologies, the accurate prediction of volcanic eruptions has significantly progressed through the analysis of ash particles and seismic data. AI is trained to recognize ash particles to identify types of volcanoes and predict eruptions accurately. IBM's Watson utilizes seismic sensors and geological data to predict volcanic eruptions with high precision. AI applications play a crucial role in preventing the loss of life in areas near active volcanoes through early eruption warnings. Scientists are actively developing AI techniques to enhance volcanic hazard prediction and mitigation strategies. By harnessing AI, experts aim to create effective mitigation techniques for volcanic hazards, ultimately improving overall disaster preparedness and response. Despite these advancements, the complex nature of volcanic systems and the unpredictability of eruptions remain challenging factors. Continued research and technological advancements are crucial in refining predictive models and enhancing early warning systems for volcanic activity.
Technology for Volcano Monitoring
The advancement of technology for volcano monitoring has revolutionized the field of predicting volcanic eruptions, offering unprecedented insights into volcanic activity and enhancing early warning capabilities. AI technology, with its capability to recognize ash particles and identify different types of volcanoes, has become instrumental in volcanic hazard prediction. IBM's Watson, for example, utilizes seismic sensors and geological data to accurately forecast volcanic eruptions, playing a crucial role in preventing the loss of life in areas near active volcanoes. Scientists are continuously developing advanced AI techniques to improve volcanic hazard prediction and create innovative mitigation strategies aimed at reducing the impact of volcanic hazards on communities. By integrating AI applications into volcano monitoring, experts can work towards more effective early warning systems and proactive measures to mitigate the risks posed by volcanic eruptions.
Tracking Hurricane Paths
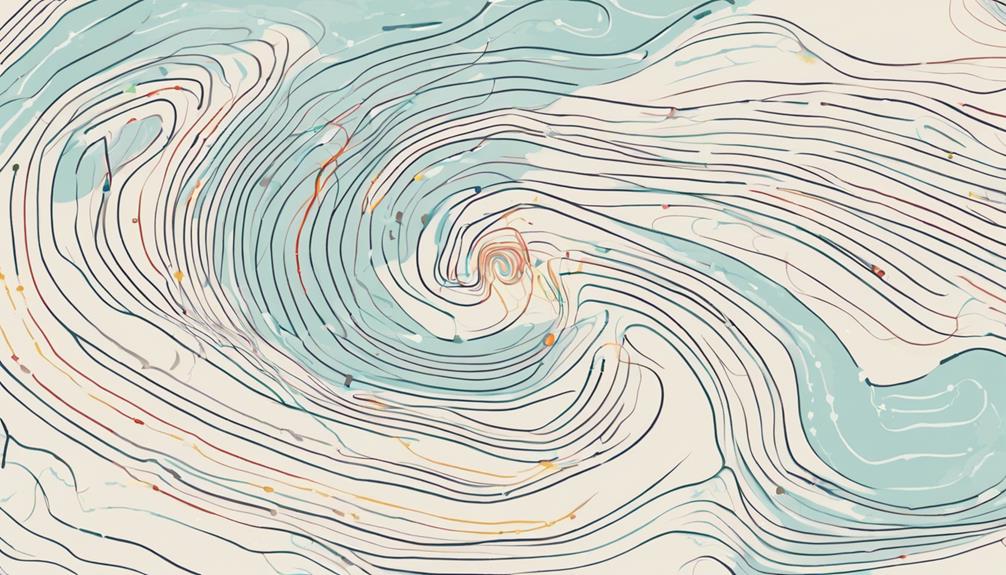
Utilizing path prediction models and data from satellites, tracking hurricane paths has become more accurate and efficient. However, questions remain regarding the reliability and applicability of these advanced technologies in real-time scenarios. Improving accuracy rates in predicting hurricane paths is crucial for enhancing preparedness and response strategies.
Path Prediction Models
With the advancement of AI technologies and machine learning algorithms, tracking hurricane paths has significantly improved in accuracy and efficiency.
- AI analyzes satellite images to predict hurricane trajectories.
- NASA and Development Seed utilize machine learning for real-time monitoring.
- Machine learning models enhance forecasting by analyzing historical data and atmospheric conditions.
These models help in predicting the intensity and potential landfall locations of hurricanes, improving preparedness and response efforts for these natural disasters. Through the utilization of AI-driven path prediction models, the scientific community can better understand and predict the behavior of hurricanes and cyclones, ultimately leading to more effective disaster management strategies.
Data From Satellites
Advancements in satellite technology have revolutionized the tracking of hurricane paths by providing crucial real-time data for predicting the trajectory and intensity of these natural disasters. Meteorologists heavily rely on satellite data to analyze various atmospheric parameters influencing hurricanes. Through satellite imagery, meteorologists can accurately forecast the trajectory of hurricanes, enabling timely warnings for potential landfall locations. Satellite-based tracking systems have significantly enhanced the precision of predicting hurricane paths, aiding in disaster preparedness and response strategies. The data obtained from satellites plays a pivotal role in monitoring the evolution of hurricanes, allowing for better understanding of their behavior and potential impact. This technological advancement has vastly improved the ability to anticipate and prepare for the destructive forces of hurricanes.
Improving Accuracy Rates
The quest for enhancing the accuracy rates in tracking hurricane paths remains a critical focus of scientific research and technological innovation.
- AI technologies, such as machine learning, and satellite data analysis have significantly improved the precision of hurricane tracking.
- NASA and Development Seed leverage AI to monitor hurricanes using satellite imagery, thereby enhancing prediction capabilities.
- AI algorithms play a vital role in predicting storm intensities and paths, enabling more effective mitigation efforts.
Accurate tracking of hurricane paths is crucial for saving lives and reducing property damage through timely evacuations. The integration of AI into monitoring and forecasting hurricanes marks a substantial advancement in the accuracy of natural disaster prediction.
Enhancing Hurricane Predictions
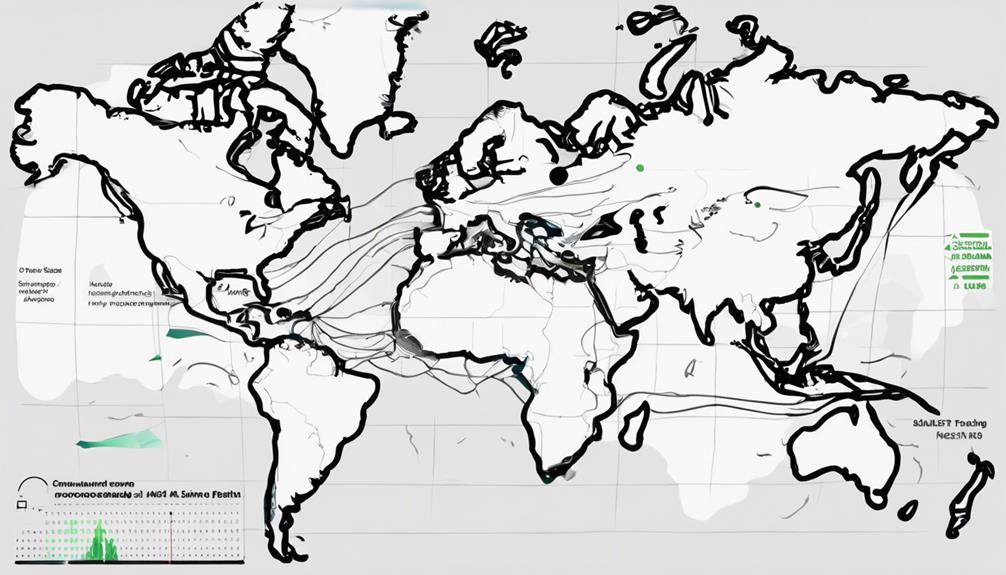
Utilizing satellite imagery and machine learning techniques, researchers and organizations are actively working to enhance the accuracy of hurricane predictions, ultimately aiming to minimize the impact of these natural disasters. By harnessing AI and analyzing vast amounts of data from satellite images, advancements in hurricane prediction capabilities have been achieved. NASA and Development Seed are at the forefront of utilizing these technologies to track hurricanes, improve path prediction accuracy, and monitor storm intensities. The collaboration between these entities and meteorological departments has led to significant progress in forecasting hurricanes, allowing for better-informed decisions regarding evacuation and preparedness measures. The integration of machine learning algorithms with satellite data enables more precise monitoring of storm trajectories, potentially saving lives and reducing property damage. Continued research and technological advancements in this field are pivotal in enhancing our ability to predict and mitigate the impact of hurricanes, highlighting the importance of ongoing efforts to refine hurricane prediction models for the safety and well-being of communities at risk.
Predictive Analytics for Wildfires
In the realm of wildfire management, the application of predictive analytics has emerged as a crucial tool for forecasting fire behavior and mitigating potential risks. Through the utilization of historical data, weather patterns, and topographical information, predictive analytics can assess the likelihood and potential spread of wildfires. Machine learning algorithms play a significant role in this process by analyzing factors such as vegetation conditions, wind speed, and humidity levels to predict wildfire behavior accurately. Additionally, the incorporation of advanced satellite imagery and drones enables real-time monitoring of wildfires, facilitating early detection and response efforts. By integrating AI models, predictive analytics can pinpoint wildfire risk areas, allowing for proactive measures like controlled burns and evacuation planning. This proactive approach not only enhances emergency preparedness but also aids in resource allocation and the development of effective firefighting strategies.
Improving Fire Risk Assessments

Considering the evolving landscape of wildfire management and the potential impact of predictive analytics, a critical focus now lies in enhancing fire risk assessments to further refine proactive measures and response strategies. AI algorithms play a pivotal role in this improvement by analyzing historical fire data to accurately predict future fire risks. Machine learning models are instrumental in assessing environmental factors to pinpoint fire-prone areas, enabling authorities to prioritize mitigation efforts effectively. Additionally, remote sensing technology aids in monitoring vegetation changes, providing valuable insights for fire risk assessments.
Role of AI in Disaster Prediction
AI's role in disaster prediction is pivotal, as it harnesses data from various sources to monitor environmental changes and forecast natural disasters. Utilizing machine learning algorithms, AI can analyze seismic data patterns, historical disaster records, and real-time information to enhance prediction accuracy. In disaster management, AI contributes significantly to early warning systems, enabling timely alerts to at-risk communities based on sophisticated predictive models. Moreover, through social media analysis, AI aids in monitoring disaster-related information, improving situational awareness for effective disaster response strategies.
Key Points:
- AI analyzes seismic data patterns to predict natural disasters accurately.
- Early warning systems leverage AI for timely alerts to at-risk communities.
- Social media analysis enhances real-time disaster information monitoring for improved disaster response.
Utilizing Machine Learning Models
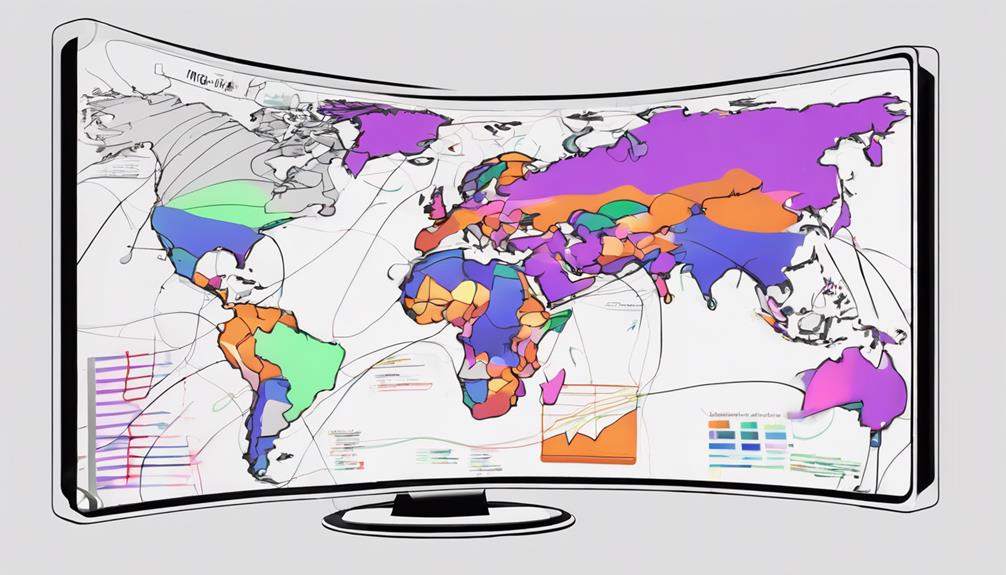
Utilizing advanced machine learning models enables researchers to analyze historical data with precision, enhancing the accuracy of predicting future natural disasters. By harnessing the power of AI, these models can process vast amounts of data from various sources to monitor environmental changes and identify patterns that precede disasters. Notably, initiatives by Google's DeepMind and Harvard have demonstrated the efficacy of AI in predicting earthquakes and rainfall, showcasing the potential of machine learning in disaster forecasting.
Moreover, AI plays a crucial role in predicting the likelihood and trajectory of wildfires, thereby improving disaster response strategies. Integrating AI with Internet of Things (IoT) devices and drones further refines real-time monitoring capabilities, allowing for swift and accurate predictions. This synergy between AI, machine learning, and disaster prediction highlights a promising avenue for enhancing preparedness and mitigating the impact of natural disasters through data-driven insights. As technology continues to advance, leveraging these sophisticated models becomes increasingly vital in safeguarding communities from the unpredictable forces of nature.
Data Collection for Prediction Accuracy
Effective prediction of natural disasters hinges on the meticulous collection of data from diverse sources such as satellites, weather stations, seismic sensors, and historical records. To enhance prediction accuracy, data collection must be continuous and comprehensive. Key elements in data collection for prediction accuracy include:
- Seismic Data: Monitoring seismic activity is essential for predicting earthquakes and tsunamis accurately.
- AI in Natural Disaster Prediction: Utilizing AI algorithms requires high-quality data to improve the precision of disaster forecasts.
- IoT Devices for Data Collection: IoT devices play a crucial role in collecting real-time data for developing advanced prediction models.
Utilizing advanced technology like drones and remote sensing, coupled with a robust data collection framework, is imperative for improving the effectiveness of early warning systems and disaster response strategies. Ensuring data accuracy and quality is paramount in refining prediction models and enhancing the reliability of forecasting natural disasters.
Future Trends in Disaster Forecasting

The evolution of disaster forecasting methodologies is increasingly reliant on the integration of advanced technologies and data-driven approaches to enhance prediction accuracy and response efficiency. AI integration with IoT devices and drones is poised to revolutionize real-time disaster response and forecasting capabilities. Evolving AI algorithms hold the promise of significantly increasing prediction accuracy and efficiency in disaster forecasting by analyzing seismic data and other critical information. Advanced AI technologies are set to automate post-disaster damage assessment through the utilization of satellite imagery, enabling rapid response and resource allocation. Moreover, the implementation of personalized alerts tailored to individual requirements will enhance communication and preparedness for natural disasters. By leveraging AI-driven simulations and modeling, disaster management authorities can develop more effective strategies to mitigate the impact of future events. These advancements in AI integration and data analytics are paving the way for a more proactive and informed approach to disaster forecasting and response.
Frequently Asked Questions
Is It Possible to Predict a Natural Disaster?
Predicting natural disasters accurately remains a challenging endeavor due to the inherent unpredictability of these events. Despite advancements in data analysis, risk assessment, and technology integration, factors such as climate patterns, geographical influences, and sudden changes in conditions can hinder precise predictions. Early warning systems and efficient emergency response mechanisms are essential for mitigating disaster impacts, highlighting the ongoing need for improved forecasting methods.
Will AI Be Able to Predict Natural Disasters?
While AI has made strides in disaster prediction, its limitations in data accuracy challenge its effectiveness. Technological advancements in machine learning have improved predictive models, aiding disaster response. However, forecasting accuracy still faces hurdles due to incomplete data sets and evolving algorithms. The integration of AI with IoT and drones enhances real-time monitoring, yet the road to precise natural disaster prediction remains complex and ongoing.
What Natural Disasters Cannot Be Predicted?
Unpredictable events such as earthquakes, volcanic eruptions, tsunamis, landslides, and tornadoes pose significant challenges in accurate forecasting due to the inherent uncertainties, unknown variables, and complex interactions within dynamic environments. These natural disasters often occur suddenly, making it difficult to predict their timing, location, magnitude, path, and intensity with precision. Forecasting limitations result in unforeseeable consequences, highlighting the complexities of predicting these events with absolute accuracy.
Which Method Is Used to Predict Natural Disasters?
Various methods are utilized to predict natural disasters, including analyzing weather patterns, satellite data, seismic activity, and employing early warning systems. Through risk assessment, climate modeling, and forecast accuracy, researchers aim to enhance predictive capabilities. These approaches rely on a combination of historical data and real-time information to improve the accuracy of natural disaster predictions.
Conclusion
Despite advancements in AI technology, the accuracy of predicting natural disasters remains questionable. While machine learning models and real-time data analysis offer hope, limitations such as data availability and unpredictable environmental changes continue to challenge the reliability of disaster forecasts. As we strive for better predictive algorithms and integration of emerging technologies, the elusive nature of accurately predicting natural disasters reminds us of the unpredictable forces of nature that ultimately defy human control.